Happy World Soils Day!
Since the last release of the Open Soil Spectral Library (OSSL), a lot of work has been done behind the scenes for updating, standardizing and harmonizing spectra and laboratory data from multiple public spectral libraries (Figure 1).
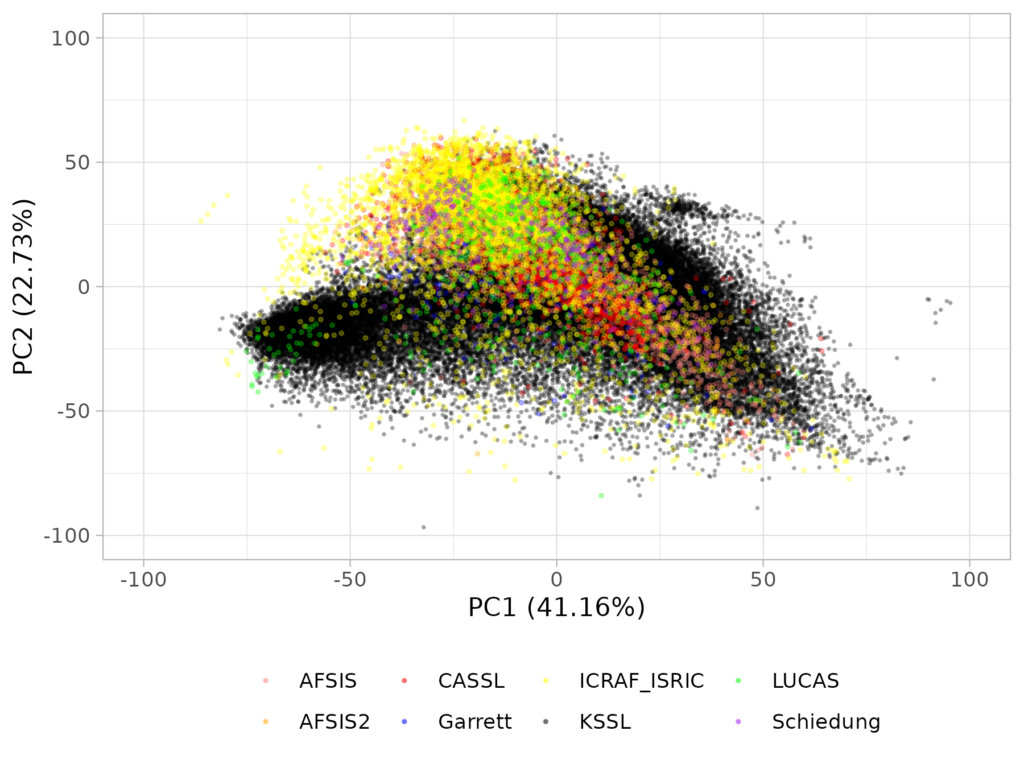
The first version included the USDA NRCS NCSS-KSSL MIR & VNIR libraries, ICRAF-ISRIC MIR & VNIR libraries, AFSIS I & AFSIS II MIR libraries, ESDAC LUCAS VNIR library, and the ETH Congo Basin MIR library (labeled CASSL in Figure 1). This new update incorporates two new datasets spanning other regions that were not geographically well-represented in the first OSSL version: The Scion Research NZ MIR library (labeled Garrett in Figure 1), and the University of Zurich permafrost MIR library (labeled Schiedung in Figure 1). In addition, as the KSSL MIR spectral library keeps growing in size and potentially represents more diverse soil types, we received a copy of the database snapshot from July 2022 for this new update (Table 1).
Table 1. Size contribution of each soil spectral library to the OSSL.
Dataset | MIR sample size | VNIR sample size |
AFSIS | 1904 | – |
AFSIS2 | 394 | – |
CASSL | 1578 | – |
Garrett | 184 | – |
ICRAF_ISRIC | 4153 | 4438 |
KSSL | 82862 | 19807 |
LUCAS | 589* | 40818 |
Schiedung | 271 | – |
Total | 91935 | 65063 |
*Some samples from the ESDAC LUCAS were scanned at Woodwell Climate Research Center for the MIR spectral range. Note: not all scans have full associated laboratory data.
Late October of this year we had an internal meeting with colleagues from University of Florida and OpenGeoHub held in place at Woodwell Climate Research Center for discussing major improvements and new directions for the OSSL database and engine. Harmonizing and importing the spectral measurements has been a time consuming but priority task since the first release of the database.
Given that some variation in spectra across different instruments were found in the inter-laboratory spectroscopy ring trial, a parallel project developed under the SoilSpec4GG initiative, our plan, in the future, is to also integrate some findings from that study to the OSSL database. This will help reduce potential mismatch between instruments and standard operating procedures (SOP) that each dataset has used before fitting our prediction models; hopefully, leading to improved and more stable model performance.
The contrasting methods used for analytically determining (wet chemistry) a given soil property has also been a subject of internal discussion in our group. Some global initiatives have been facing this same issue in their soil databases but there still no clear or full consensus on how to harmonize those different methods. This has been a topic of great discussion and research development at the Global Soil Partnership’s Global Soil Laboratory Network (GLOSOLAN).
In order to maximize transparency, for now, we have decided to produce two different levels for the OSSL database. Level 0 takes into account the original methods employed in each dataset but tries to initially fit them to our reference lists: KSSL Guidance – Laboratory Methods and Manuals and ISO standards. If a reference method does not fall in any previous method, then we create a new variable sharing at least a common property and unit. A final harmonization takes place in the OSSL Level 1, where those common properties sharing different methods can be converted to a target method using some publicly available transformation rule, or in the worst scenario, they are naively binded or kept separated to produce its specific model. All the implementations will be documented in our processing github repository.
Another interesting update that we are keeping in mind but depends on the database update is the review of our prediction models deployed on the OSSL engine. Right now, we have received important feedback from people that said the current models are doing a great job, but for others not that much. This can be due to the specific soil types not being well represented or due to the spectra not being well aligned with the OSSL. We plan to conduct a systematic analysis of learning algorithms, global vs local fitting, compression methods and preprocessing using the OSSL database. Another great interest is to test if including spatial covariates or fusing different spectral regions really help to deliver more reliable predictions. We are happy that this topic was recently explored in a paper of global prediction of soil salinity where the OSSL has enabled new science.
We will send out an update note once the Level 0 and Level 1 databases are uploaded into the OSSL. It will be before the end of the year.